Notice
Recent Posts
Recent Comments
Link
일 | 월 | 화 | 수 | 목 | 금 | 토 |
---|---|---|---|---|---|---|
1 | ||||||
2 | 3 | 4 | 5 | 6 | 7 | 8 |
9 | 10 | 11 | 12 | 13 | 14 | 15 |
16 | 17 | 18 | 19 | 20 | 21 | 22 |
23 | 24 | 25 | 26 | 27 | 28 |
Tags
- 파이썬
- Algorithm
- 가상환경
- 프로그래머스
- 노마드코딩
- String Method
- aws jupyter notebook
- queue
- MySQL
- 백준
- javascript
- 알고리즘스터디
- Matplotlib
- Join
- python
- 알고리즘
- 코딩테스트
- type hint
- 정보처리기사 c언어
- Stack
- 선그래프
- 알고리즘 스터디
- 자료구조
- Selenium
- 데이터시각화
- pandas
- openCV
- dataframe
- programmers
- NumPy
Archives
- Today
- Total
조금씩 꾸준히 완성을 향해
[OpenCV/Python] 임계값(Threshold) 처리 (BINARY, BINARY_INV, TRIANGLE, OTSU 등) 본문
Python/OpenCV
[OpenCV/Python] 임계값(Threshold) 처리 (BINARY, BINARY_INV, TRIANGLE, OTSU 등)
all_sound 2022. 11. 12. 23:23임계값 처리 (Threshold)
- cv2.THRESH_BINARY
- cv2.THRESH_BINARY_INV
- cv2.THRESH_TRUNC
- cv2.THRESH_TOZERO
- cv2.THRESH_TOZERO_INV
Parameters
src | input array (multiple-channel, 8-bit or 32-bit floating point). |
dst | output array of the same size and type and the same number of channels as src. |
thresh | threshold value. |
maxval | maximum value to use with the THRESH_BINARY and THRESH_BINARY_INV thresholding types. |
type | thresholding type. |
# lena image
src = cv2.imread('./lena.jpg', cv2.IMREAD_GRAYSCALE)
ret, dst = cv2.threshold(src, 100, 255, cv2.THRESH_BINARY) #threshold=100, THRESH_BINARY
print('ret=', ret)
cv2.imshow('src', src)
cv2.imshow('dst', dst)
cv2.waitKey()
cv2.destroyAllWindows()
# ret= 100.0
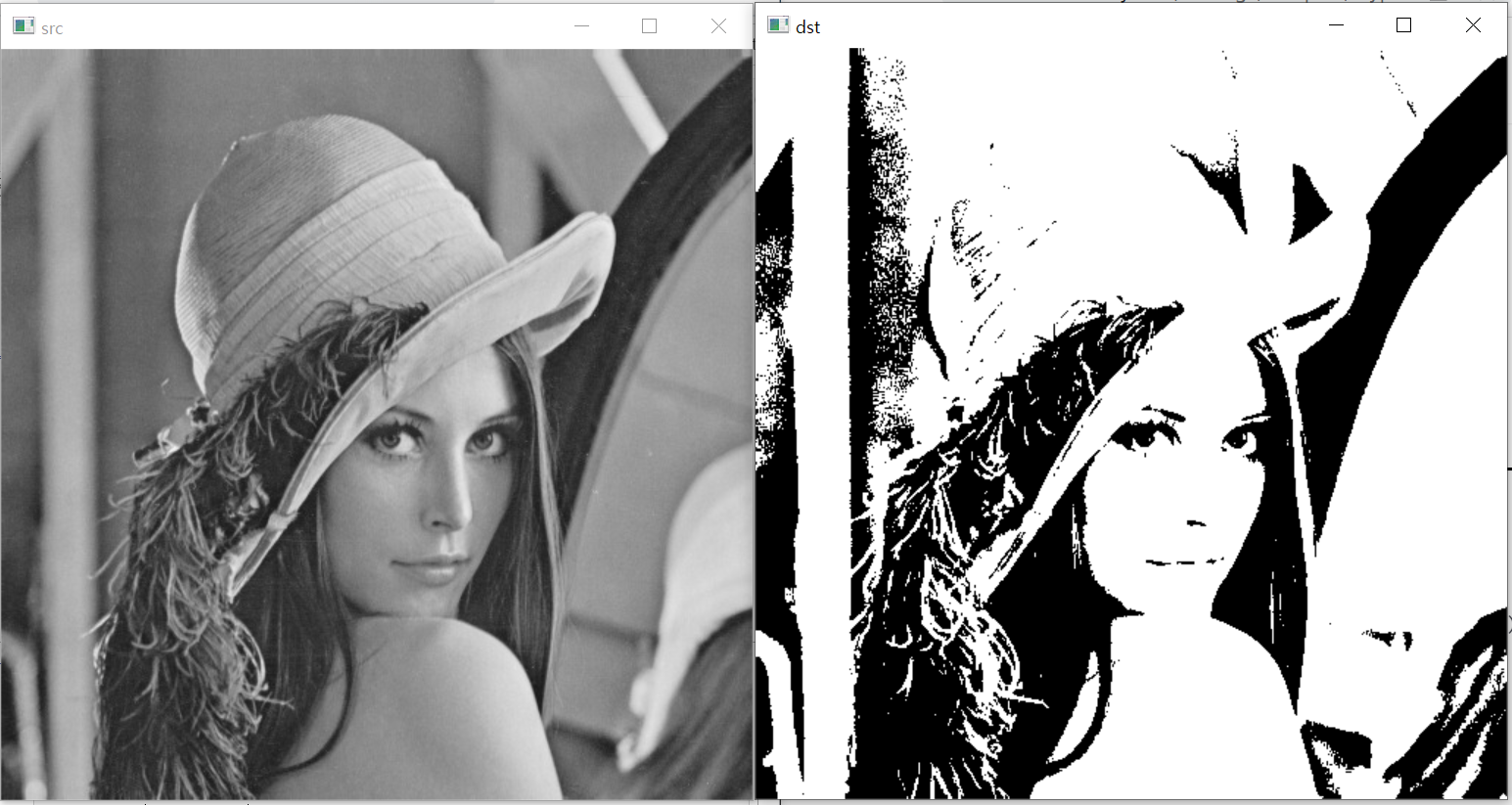
# THRESH_BINARY vs THRESH_BINARY_INV
# cup image
src = cv2.imread('./cup.png', cv2.IMREAD_GRAYSCALE)
ret1, dst1 = cv2.threshold(src, 183, 255, cv2.THRESH_BINARY) #threshold=183
ret2, dst2 = cv2.threshold(src, 180, 255, cv2.THRESH_BINARY_INV) #threshold=180
cv2.imshow('src', src)
cv2.imshow('dst1', dst1)
cv2.imshow('dst2', dst2)
cv2.waitKey()
cv2.destroyAllWindows()
# THRESH_TRUNC vs THRESH_TOZERO vs THRESH_TOZERO_INV
src = cv2.imread('./cup.png', cv2.IMREAD_GRAYSCALE)
ret1, dst1 = cv2.threshold(src, 180, 255, cv2.THRESH_TRUNC)
ret2, dst2 = cv2.threshold(src, 180, 255, cv2.THRESH_TOZERO)
ret3, dst3 = cv2.threshold(src, 180, 255, cv2.THRESH_TOZERO_INV)
cv2.imshow('dst1', dst1)
cv2.imshow('dst2', dst2)
cv2.imshow('dst3', dst3)
cv2.waitKey()
cv2.destroyAllWindows()
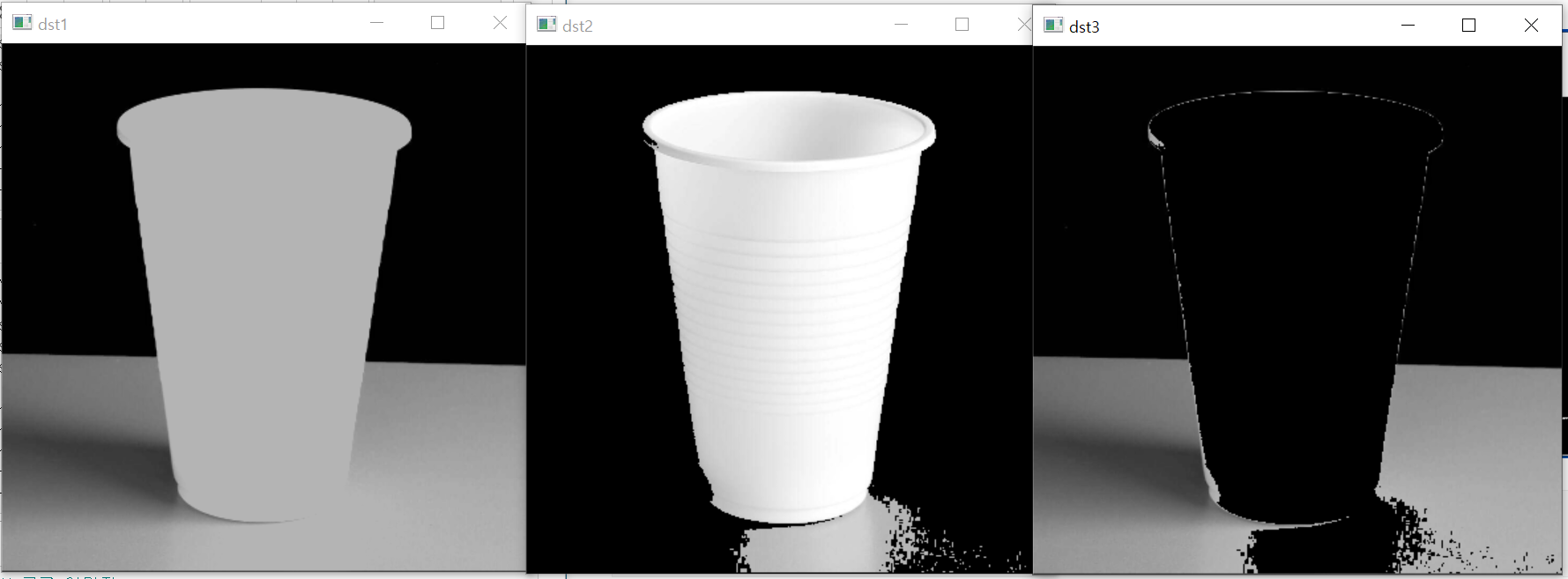
# openCV 로고 이미지
src = cv2.imread('./opencv_logo.png', cv2.IMREAD_GRAYSCALE)
ret, dst = cv2.threshold(src, 240, 255, cv2.THRESH_BINARY)
cv2.imshow('src', src)
cv2.imshow('dst', dst)
cv2.waitKey()
cv2.destroyAllWindows()
# 흰 배경에 있는 도형들이라 구분이 잘 됨
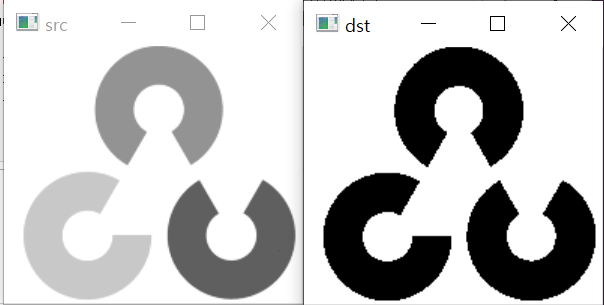
# 정규화 (normarlization)
- minMaxLoc() : 최대값, 최소값, 최대값 위치, 최소값 위치 반환
# minMaxLoc
src = cv2.imread('./lena.jpg', 0)
minVal, maxVal, minLoc, maxLoc = cv2.minMaxLoc(src)
print('src')
print('minVal:', minVal)
print('maxVal:', maxVal)
print('minLoc:', minLoc)
print('maxLoc:', maxLoc)
print('src[minLoc[1], minLoc[0]]:', src[minLoc[1], minLoc[0]])
print('src[maxLoc[1], maxLoc[0]]:', src[maxLoc[1], maxLoc[0]])
# src
# minVal: 18.0
# maxVal: 247.0
# minLoc: (265, 198)
# maxLoc: (116, 273)
# src[minLoc[1], minLoc[0]]: 18
# src[maxLoc[1], maxLoc[0]]: 247
- normalize
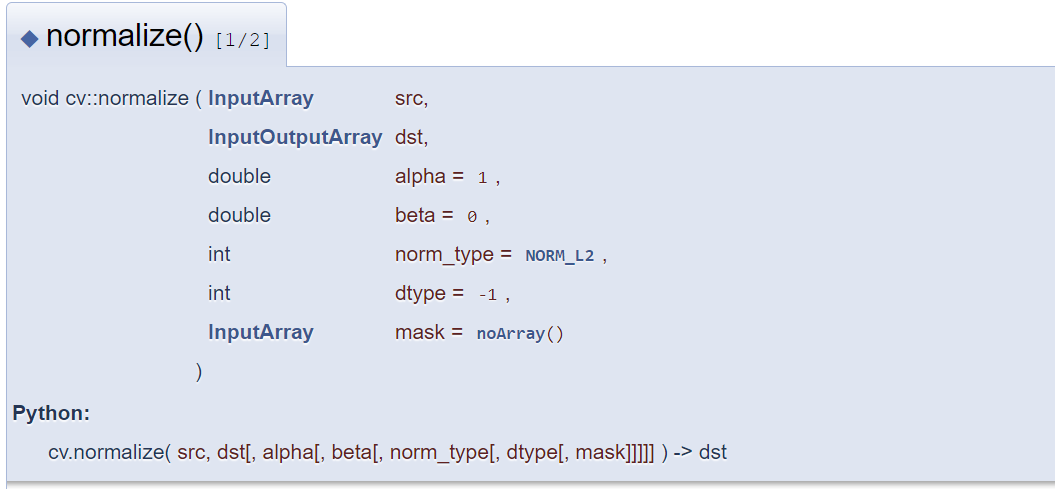
Parameters
src | input array. |
dst | output array of the same size as src . |
alpha | norm value to normalize to or the lower range boundary in case of the range normalization. |
beta | upper range boundary in case of the range normalization; it is not used for the norm normalization. |
norm_type | normalization type . |
dtype | when negative, the output array has the same type as src; otherwise, it has the same number of channels as src and the depth =CV_MAT_DEPTH(dtype). |
mask | optional operation mask. |
# normalize
dst = cv2.normalize(src, None, 0, 255, cv2.NORM_MINMAX)
minVal, maxVal, minLoc, maxLoc = cv2.minMaxLoc(dst)
print('src')
print('minVal:', minVal)
print('maxVal:', maxVal)
print('minLoc:', minLoc)
print('maxLoc:', maxLoc)
print('src[minLoc[1], minLoc[0]]:', src[minLoc[1], minLoc[0]])
print('src[maxLoc[1], maxLoc[0]]:', src[maxLoc[1], maxLoc[0]])
# src
# minVal: 0.0
# maxVal: 255.0
# minLoc: (265, 198)
# maxLoc: (116, 273)
# src[minLoc[1], minLoc[0]]: 18
# src[maxLoc[1], maxLoc[0]]: 247
# 이미지 확인
cv2.imshow('src', src)
cv2.imshow('dst', dst)
cv2.waitKey()
cv2.destroyAllWindows()
# 조금 더 선명해짐 (밝기 대비가 강해짐)
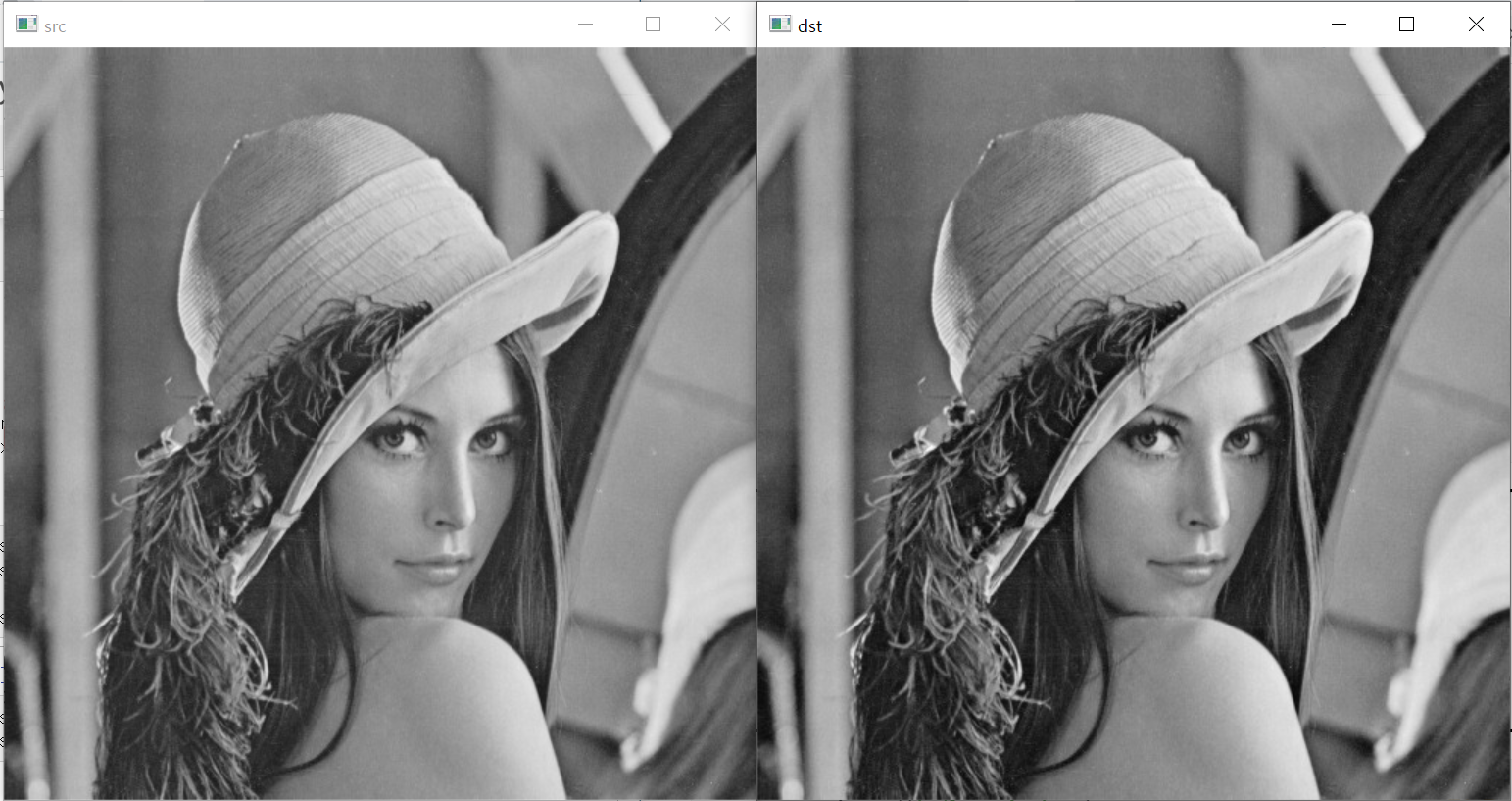
- 0~255까지 정규화 직접 만들어서 이미지 구현
src = cv2.imread('./lena.jpg', 0)
print('min:', src.min()) # min: 18
print('max:',src.max()) # max: 247
dst = (src - np.min(src)) / (np.max(src)-np.min(src))*255 #정규화
print('min:',dst.min()) # min: 0.0
print('max:',dst.max()) # max: 255.0
# 결과 확인
cv2.imshow('src', src)
cv2.imshow('dst', np.uint8(dst))
cv2.waitKey()
cv2.destroyAllWindows()
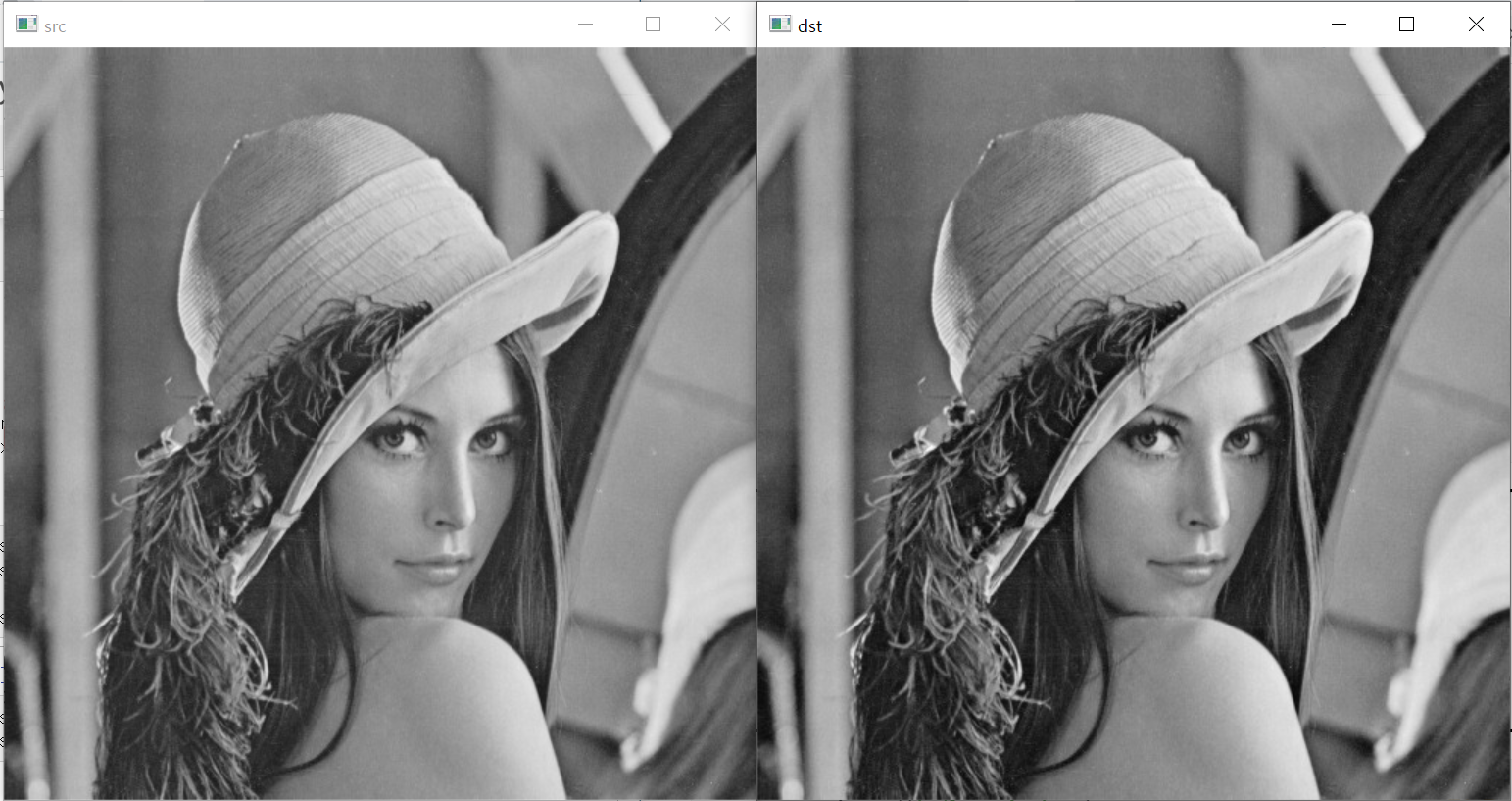
# 자동 임계값 계산
- cv2.THRESH_TRIANGLE : 삼각 알고리즘을 이용한 자동 임계값 결정
- cv2.THRESH_OTSU : 오츠 알고리즘을 이용한 자동 임계값 결정
# lena image
src = cv2.imread('./lena.jpg', 0)
ret, dst = cv2.threshold(src, 200, 255, cv2.THRESH_BINARY)
ret1, dst1 = cv2.threshold(src, 200, 255, cv2.THRESH_BINARY+cv2.THRESH_TRIANGLE)
ret2, dst2 = cv2.threshold(src, 200, 255, cv2.THRESH_BINARY+cv2.THRESH_OTSU)
print('threshold=', ret)
print('threshold_triangle=', ret)
print('threshold_otsu=', ret2)
# threshold= 200.0
# threshold_triangle= 200.0
# threshold_otsu= 117.0
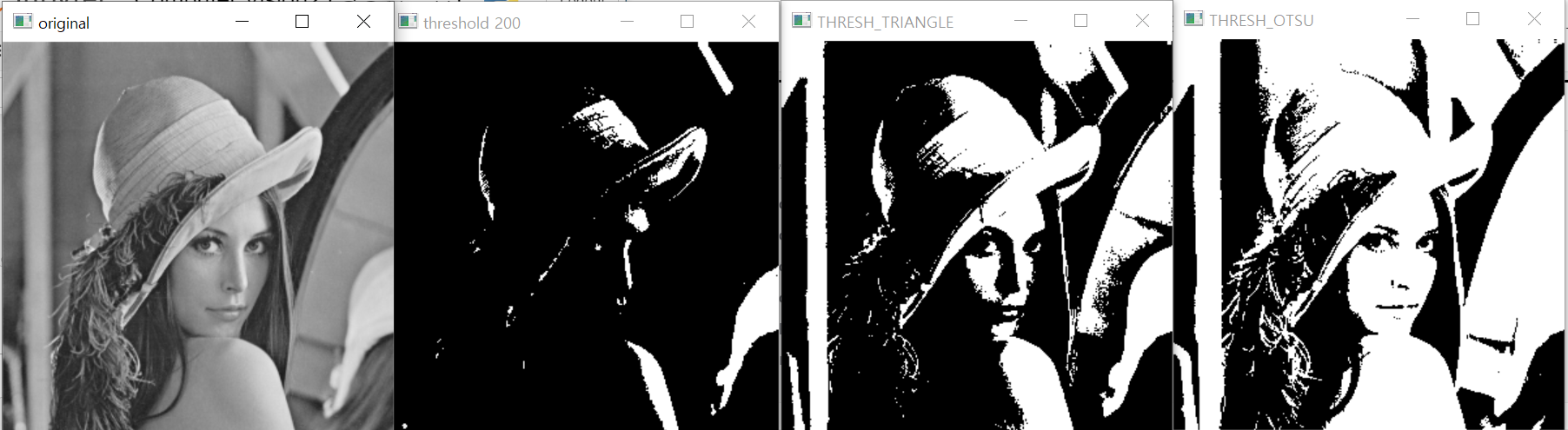
# cup image
src = cv2.imread('./cup.png', 0)
src = cv2.resize(src, dsize=(300,300))
ret, dst = cv2.threshold(src, 200, 255, cv2.THRESH_BINARY)
ret1, dst1 = cv2.threshold(src, 200, 255, cv2.THRESH_BINARY+cv2.THRESH_TRIANGLE)
ret2, dst2 = cv2.threshold(src, 200, 255, cv2.THRESH_BINARY+cv2.THRESH_OTSU)
print('threshold=', ret)
print('threshold_triangle=', ret)
print('threshold_otsu=', ret2)
# threshold= 200.0
# threshold_triangle= 200.0
# threshold_otsu= 106.0
# 결과 비교
cv2.imshow('original', src)
cv2.imshow('threshold 200', dst)
cv2.imshow('THRESH_TRIANGLE', dst1)
cv2.imshow('THRESH_OTSU', dst2)
cv2.waitKey()
cv2.destroyAllWindows()
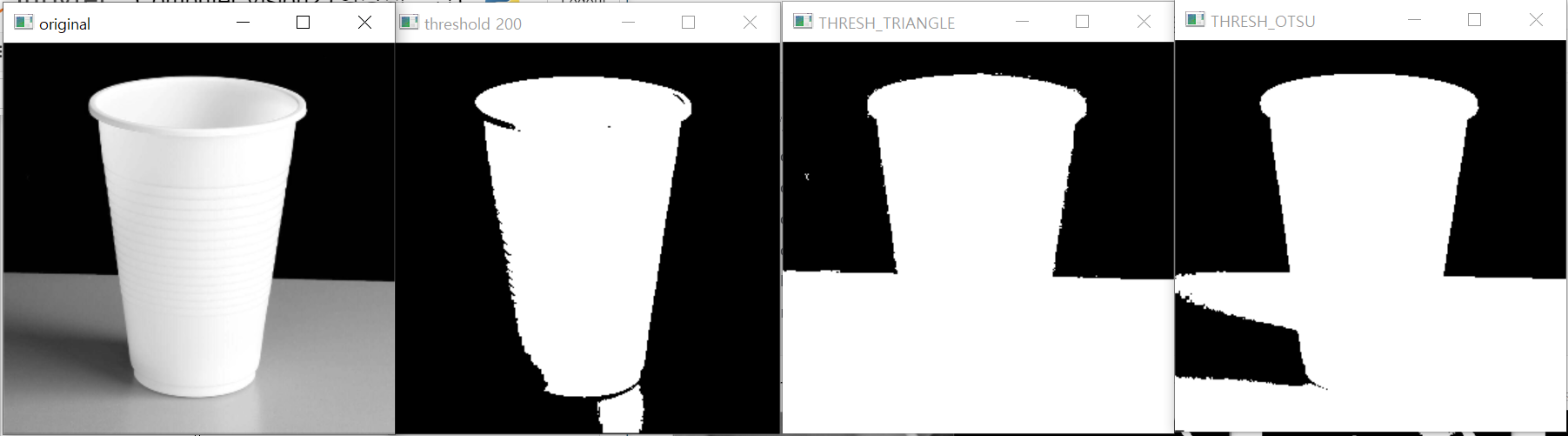
- cv2.ADAPTIVE_THRESH_MEAN_C
- cv2.ADAPTIVE_THRESH_GAUSSIAN_C
# lena
src = cv2.imread('./lena.jpg', 0)
ret, dst = cv2.threshold(src, 200, 255, cv2.THRESH_BINARY)
ret1, dst1 = cv2.threshold(src, 200, 255, cv2.THRESH_BINARY+cv2.THRESH_OTSU)
print('global threshold=', ret1)
# global threshold= 117.0
dst2 = cv2.adaptiveThreshold(src, 255, cv2.ADAPTIVE_THRESH_MEAN_C, cv2.THRESH_BINARY, 51, 0)
dst3 = cv2.adaptiveThreshold(src, 255, cv2.ADAPTIVE_THRESH_GAUSSIAN_C, cv2.THRESH_BINARY, 51, 0)
cv2.imshow('threshold 200', dst)
cv2.imshow('THRESH_OTSU', dst1)
cv2.imshow('ADAPTIVE_THRESH_MEAN_C', dst2)
cv2.imshow('ADAPTIVE_THRESH_GAUSSIAN_C', dst3)
cv2.waitKey()
cv2.destroyAllWindows()
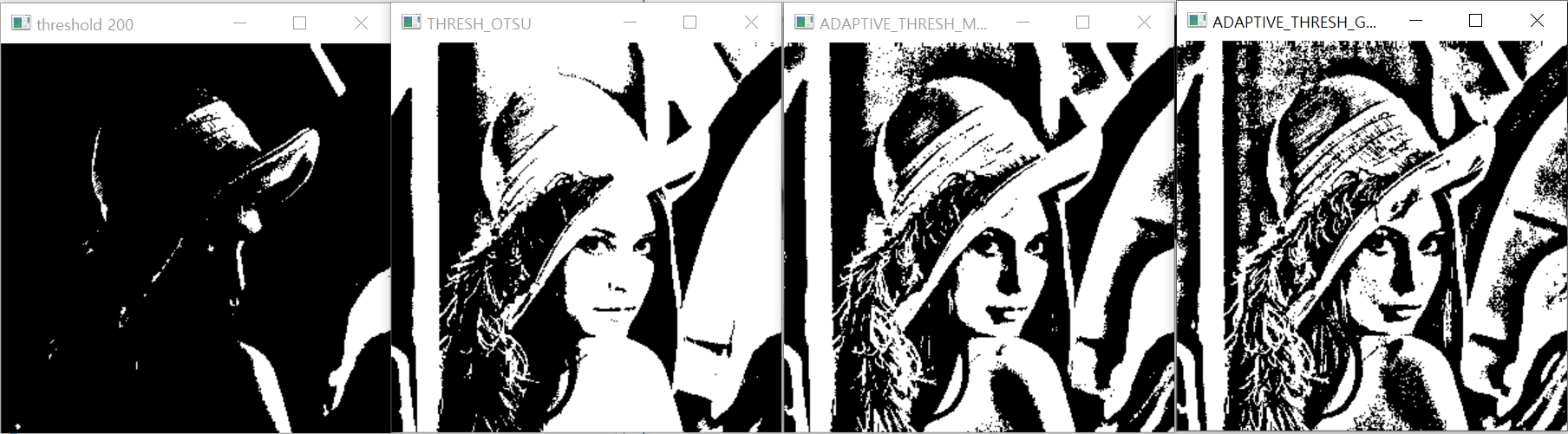
# for문 사용해서 최적의 임계값 찾기
import matplotlib.pyplot as plt
src = cv2.imread('./cup.png', 0)
dst_list = []
size_list = []
fig = plt.figure(figsize=(30, 40))
for i in range(21, 182, 20):
for j in range(21, 102, 10):
dst_list.append(cv2.adaptiveThreshold(src, 255, cv2.ADAPTIVE_THRESH_MEAN_C, cv2.THRESH_BINARY, i, j))
size_list.append([i, j])
# dst_list.append(cv2.adaptiveThreshold(src, 255, cv2.ADAPTIVE_THRESH_GAUSSIAN_C, cv2.THRESH_BINARY, i, j))
for i in range(1, 82):
ax = fig.add_subplot(9, 9, i)
ax.imshow(dst_list[i-1], 'gray')
ax.set_title(size_list[i-1])
plt.show()
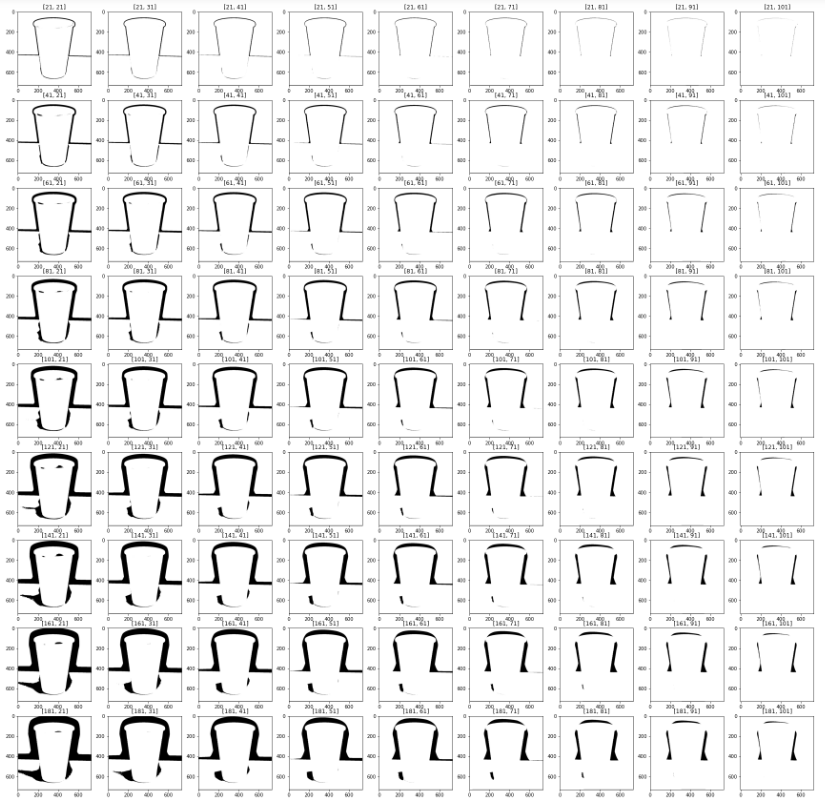
# 4가지 방법 비교
cup1=cv2.imread('./cup.png',0)
ret1,cup1=cv2.threshold(cup1,175,255,cv2.THRESH_BINARY)
ret4,cup4=cv2.threshold(cup,200,255,cv2.THRESH_BINARY+cv2.THRESH_OTSU)
print('global threshold=',ret4)
# global threshold= 106.0
cup3=cv2.adaptiveThreshold(cup,255,cv2.ADAPTIVE_THRESH_MEAN_C,cv2.THRESH_BINARY,31,21)
cup4=cv2.adaptiveThreshold(cup,255,cv2.ADAPTIVE_THRESH_GAUSSIAN_C,cv2.THRESH_BINARY,31,21)
titles=['binary','binary+otsu','mean','gaussian']
images = [cup1,cup2,cup3,cup4]
for i in range(4):
plt.subplot(2,2,i+1)
plt.imshow(images[i],'gray')
plt.title(titles[i])
plt.xticks([]),plt.yticks([])
plt.show()
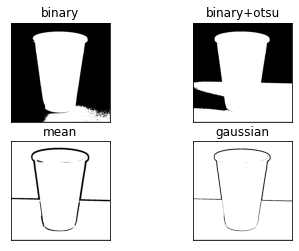